近日,js金沙3983总站胡雪梅教授和指导的统计学硕士研究生杨俊文合作完成的论文“Group penalized multinomial logit models and stock return direction prediction”被IEEE Transactions on Information Theory(IEEE TIT)在线发表。IEEE TIT期刊是中国计算机学会(CCF)推荐的A类期刊,是国际公认的理论计算机科学与信息论领域的顶级期刊,被很多人认为是工程学理论方向的天花板,享有卓越的学术声誉。这是js金沙3983总站师生首次在该期刊发表论文。
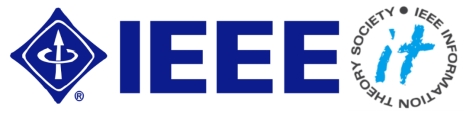
论文链接:
https://ieeexplore.ieee.org/document/10471617
论文题目:Group penalized multinomial logit models and stock return direction prediction(组惩罚多项logit模型和股票回报方向预测)
作者:胡雪梅、杨俊文
通讯作者:胡雪梅
论文摘要:Multinomial logit model(MLM) has been proposed as the most frequently regression model for multi-category response.To deal with correlated data, in this paper we propose G-LASSO/G-SCAD/G-MCP penalized MLM to exert class discovery and class prediction for high-dimensional multi-category classification problems. Firstly, we develop a group coordinate descent(GCD) algorithm to simultaneously complete group selection and group estimation, and prove the convergence to the proposed GCD algorithm under mild conditions. Secondly, we apply the training set and group estimations to obtain class probability estimators, choose the Bayes classifier to identify class index information, and introduce the testing set and a few measures to assess multi-category prediction performance. Simulations show that the proposed methods outperform LASSO/SCAD/MCP penalized MLM, 3 deep learning methods and 3 machine learning methods in terms of Kappa, PDI(polytomous discrimination index), Optimal or Average Accuracy. Finally, we combine group penalized MLM with 58 technical indicators to predict up trends, sideways trends and down trends for stock returns, and show that the proposed methods outperform the other 9 methods in terms of Accuracy, PDI, Kappa and HUM. Therefore, the proposed method can not only accommodate the correlation information, but also improve multi-category prediction performance by shrinking group coefficients.